A major problem in the treatment of AIDS is the development of drug resistance by the human immuno-deficiency virus (HIV). HIV-1 protease is the enzyme that is crucial to the role of the maturation of the virus and is therefore an attractive target for HIV/AIDS therapy. Although several effective treatment regimes have been devised which involve inhibitors that target several viral proteins 11, the emergence of drug resistant mutations in these proteins is a contributing factor to the eventual failure of treatment.
Doctors have limited ways of matching a drug to the unique profile of the virus as it mutates in each patient. A drug treatment regimen is prescribed using knowledge-based clinical decision support software, which attempts to determine optimal inhibitors using existing clinical records of treatment response to various mutational strains. The patient’s immune response is used as a gauge of the drug's effectiveness and is periodically monitored so that ineffective treatment can be minimised through an appropriate change in the regimen. The FP6 EU project ‘Virolab’ is attempting to enhance the efficacy of clinical decision support software, through a unification of existing databases, as well as integration with means of assessing drug resistance at the molecular level 12.
At the molecular level, it is the biochemical binding affinity (free energy) with which an inhibitor binds to a protein target that determines its efficacy. Experimental methods for determining biomolecular binding affinities are well established and have been implemented to study the in-vitro resistance conferred by particular mutations. These in turn add invaluable information to any decision support system, but are limited as studies are performed usually on key characteristic mutations and not with respect to the unique viral sequence of a patient. An exhaustive experimental determination of drug binding affinities in a patient-specific approach is far too costly and time-consuming to perform in any clinically relevant way.
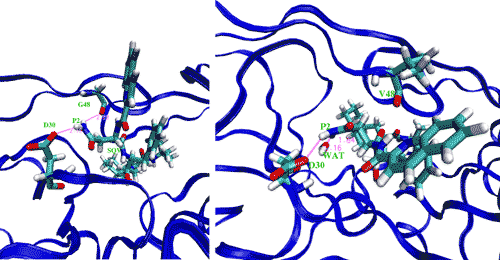
Computational methods also exist for determining biomolecular binding affinities. In a recent study 13, the effectiveness of the drug saquinavir was tested against the wildtype HIV-1 protease, along with three drug-resistant strains using free energy methods in molecular dynamics (MD) simulations (Figure 3). The protocol implemented by the study gave accurate correlations to similar experimentally determined binding affinities. Furthermore, the study made use of a tool, the Binding Affinity Calculator (BAC), for the rapid and automated construction, deployment, implementation and post processing stages of the molecular simulations across multiple supercomputing, grid-based resources. The BAC is built on top of the Application Hosting Environment (AHE) 14, a web services environment designed to hide the complexity of application launching from the scientific end user of the grid. The AHE makes use of Globus Toolkit versions 2 and/or 4 for job submission, and GridFTP for data transfer between resources.
BAC automates binding affinity calculations for all nine drugs currently available to inhibit HIV-1 protease and for an arbitrary number of mutations away from a given wildtype sequence. Although the applicability of the method in the saquinavir-based study still needs to be established for all other inhibitors, the scope of BAC is enormous as it offers an automated in-silico method for assessing the drug resistance for any given viral strain. The turn around time using BAC for such studies is seven days (per drug/protease system) with optimal computational resources; this is more than suitable for the timescales required for effective clinical decision support. Given enough computational power such that binding affinity calculations can be routinely applied, the potential to achieve patient-specific HIV decision support may then become realistic.